October 13, 2023
10:00 AM – 12:00 PM
Lakeview Hall 1104
Contact: Brian Leventhal, leventbc@jmu.edu for more information.
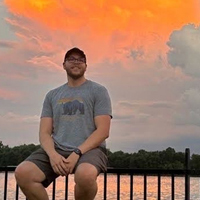
What’s the Difference: Evaluating the Validity Claims of a General Education Program
Josiah Hunsberger
General education provides students with the flexibility to tailor their college experience. However, flexibility in general education presents concerns of opportunity to learn key outcomes set forth by institutions. The current study investigates the presence of multigroup differential item functioning among different courses.
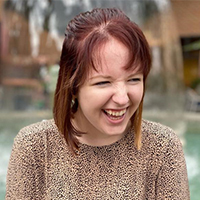
Context Matters: The Impact of External Events on Low-Stakes Assessment
Kelsey Nason & Christine DeMars
Universities use low-stakes assessment to assess general education programming and student learning objectives (Pastor et al., 2019). Low effort and low student motivation threatens the validity of aggregate scores and cross-cohort comparisons (Rios, 2021; Wise & DeMars, 2010; Yildrum-Erbasloi & Bulut, 2020). The effects of low effort and low student motivation are compounded by contextual factors of test-taking. Using time spent testing as a proxy for effort (Wise & Kong, 2005), the effort and scores of our university’s low-stakes assessment results were examined from four administrations that presented unique, changing contexts to observe the impacts of different external events. Special attention was paid to the Spring 2022 semester, which had numerous contextual factors (e.g., online assessment, suicides on campus) affecting students and their assessment environments. Time spent testing varied across semesters mirroring the varied scores. Effort on the Spring 2022 assessments was lower than all other semesters. Cross-cohort comparisons were distorted as student scores showed evidence of no growth or “learning less.” This study has implications for interpretation of test scores and cross-cohort comparisons in the face of contextual factors.
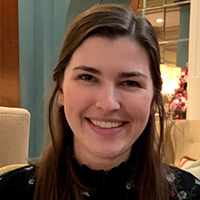
The Influence of Disengagement on the Factor Structure of a Non-Cognitive Measure: Practical Solutions
Kate Schaefer & Sara Finney
Disengaged participants are a nuisance for the interpretation of non-cognitive scale scores. Disengagement negatively influences the factor structure of scores and, in turn, the scoring of the measure (Woods, 2006). This disengagement is manifested by examinees responding too quickly (rapid response) (e.g., Wise, 2017), self-reporting low effort (e.g., Thelk et al., 2009), or consistently selecting the same response option, even though items are reverse-scored (e.g., Voss, 2023; Woods, 2006). We gathered data from 3,169 students who completed a non-cognitive measure and investigated the utility of removing data from students who streamlined (n = 141), who rapidly responded on an adjacent measure (n = 488), or who self-reported having low-effort (n = 425). We had two hypotheses: 1) there would be some overlap between examinees who displayed each type of disengagement behavior; and 2) after removing disengaged examinees, model-data fit would improve. Results supported both hypotheses. There was some overlap between examinees who used each type of disengagement behavior. Additionally, the hypothesized two-factor model fit the data better when disengaged respondents were removed via streamline filtering, rapid response filtering, or self-report filtering. Implications for the effects of disengagement on model-data fit and the practicality of selecting a motivation filtering technique are discussed.
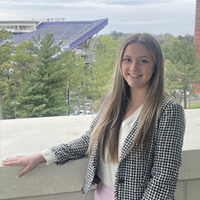
Do Multiple Doses of the Question-Behavior Effect Provide a Solution to Issues with Low Effort Later in a Testing Session?
Mara McFadden & Sara Finney
Priming examinees with questions about intended effort prior to testing has been shown to significantly increase examinee expended effort via self-reported effort and response time effort (Finney & McFadden, in press). However, this question-behavior effect seems to wear off later in a testing session, specifically when a test is given second in the session. This finding aligns with testing literature which has shown that tests and items later in a testing session tend to receive less student effort (e.g., DeMars, 2007; Wise, 2006). The current study evaluated whether administering a second “dose” of the question-behavior effect could combat the decrease in examinee effort later in a testing session. To evaluate the effectiveness of “double dosing” to increase examinee effort later in a testing session, we randomly assigned examinees to one of three question conditions prior to completing two low-stakes tests: answering three questions about intended effort directly before the first test in a session, answering three questions about intended effort directly before each test in a session, and answering no questions (control). The two tests were counter-balanced and response time was collected at the item level to gauge effort throughout the test session. Administering a second dose of questions directly before the second test in a session significantly increased examinee response-time effort for the more difficult test. Thus, this simple administration of multiple sets of questions throughout a testing session appears to combat issues with low effort on difficult tests that are administered later in a testing session.
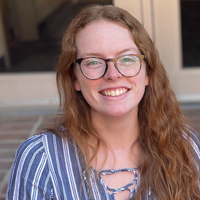
Real or fake? Connecting student learning and graduation rates across time
Autumn Wild, M.A., & Joseph M. Kush, Ph.D.
College graduation rates have dramatically increased since the 1990s. It has been questioned whether this increase is associated with student learning or a different factor (i.e., grad inflation). Using data from a public university, student learning was shown to be significantly and positively related to graduation rates.
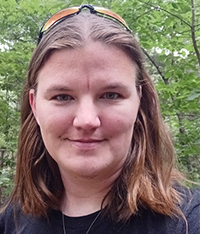
Learning Improvement in STEM: Responding to Faculty Situational Factors
Laura Lambert & Megan Good
Learning improvement projects are large, complex, time-consuming, and rarely seen in the literature. One STEM department set out to undertake a learning improvement project, learned that the data was already available to evaluate a large curricular change, allowing them to evidence learning improvement even with overwhelmed faculty.
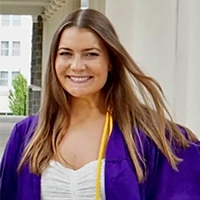
Finding Latent Profiles of Student Success Skills to Predict Retention
Chris Patterson (PhD) & Riley Herr
This paper introduces latent profile analysis as an alternative method to help predict college student retention. By using LPA on a collection of various success skills and attitudes, this paper shows that even the slightest increase or decrease in attitudes or skills can affect a student's likelihood of retention.