Is AI ready for its close-up?
News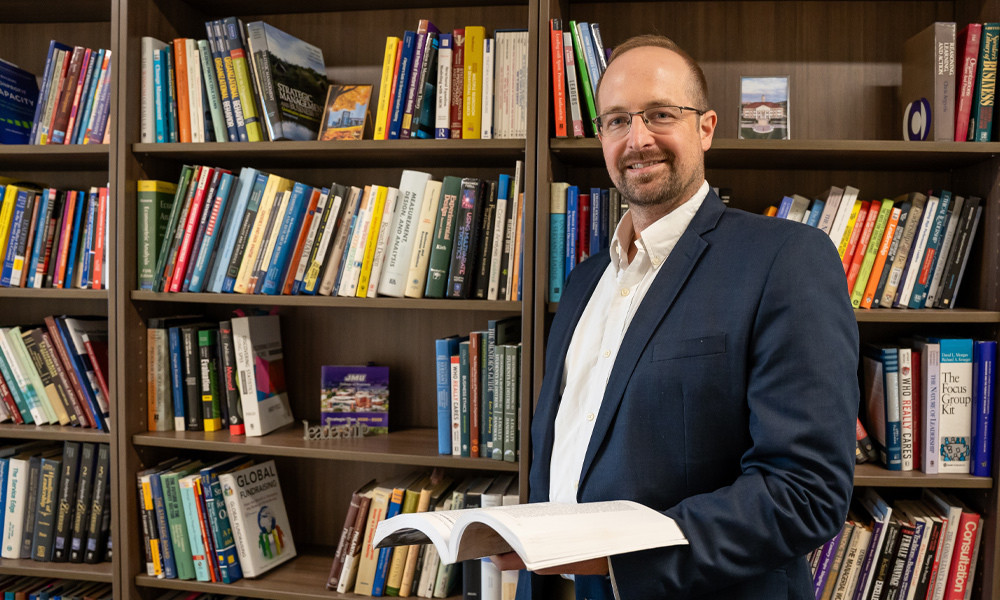
SUMMARY: Madison Scholar assesses whether robotic technologies are primed to take over human resource functions performed by humans.
Adam Vanhove, associate professor in the School of Strategic Leadership Studies' doctoral program, is the College of Business’ Madison Scholar for the current academic year.
To mark his selection for this prestigious honor, which goes to a CoB faculty member with a sustained record of outstanding scholarship, Vanhove gave a presentation on Nov. 1 of some of his more recent work.
His remarks were delivered in-person during a noontime luncheon in Zane Showker Hall, and were also shared via livestream.
Vanhove's research has garnered more than 1,100 Google Scholar citations to-date. He has also served with distinction as an editor of scholarly publications, and has cultivated strong professional relationships that have resulted in significant external funding.
Much of Vanhove’s research is concerned with human-resources management issues, specifically employee resilience and health; employment discrimination (specifically due to employee gender, race and weight); and followership and leader-follower relations.
He earned a bachelor's degree in psychology at the University of Minnesota Duluth, a master's degree in industrial-organizational psychology at Colorado State University and a doctorate in industrial-organizational psychology, also at Colorado State.
For his talk, which was titled in part, "The future of AI is here ... kind of," Vanhove looked at data on the effectiveness of supervised machine learning — a specific type of artificial intelligence — in predicting human-resources management outcomes.
Among the outcomes he assessed were employee recruitment, hiring, performance evaluation, promotion and turnover. Vanhove examined data from 74 different scientific journal articles, conference presentations and books. His statistical integration of the results of these prior studies yielded a number of interesting findings.
In the run-up to his Nov. 1 presentation, Vanhove spoke to us informally about those findings — especially as they relate to human-resources management.
College of Business: Where does this paper fit into your overall body of work?
Adam Vanhove: It's a new area of research I'm pursuing. A lot of my background is in employee well-being, and discrimination in the workplace. In popular media, this had become a very hot topic … and it seemed really interesting to me. I kept seeing one version or another of the statement, "AI is going to change the way we manage human resources." But I didn't see a lot of research in the human-resources management literature that was actually testing and validating these tools to put them into use. So I did a really thorough review of the literature in order to find out what evidence was out there. I started to find out that there was a lot … but the research wasn’t being conducted by people with expertise in human-resources management. And it wasn't appearing in the journals that human-resources managers and scholars read. So I compiled the research and summarized it for a human-resources management audience, to inform their own research going forward.
CoB: Your presentation is titled, ‘The future of AI is here … kind of.’ You seem to be alluding to some difficulties there?
Vanhove: One of the cool things about meta-analysis is that you take all of the quantitative data other studies have produced that answer a specific research question, and you aggregate it. Any one study can be fairly unreliable. But if you take 74 different studies — as we did in our meta-analysis — you can get a pretty good average indication of what exists across all those different contexts. One of the most basic things we found was that the AI — the machine-learning algorithms that are being tested to predict, for instance, whether an employee is going to leave the company in the next six months — isn’t any more effective than the human beings who are currently carrying out those functions. So AI is “here,” in the sense that we’ve started to test the use of machine learning. But the tools aren’t sufficiently effective at this point to change the way we manage human resources.
CoB: What is ‘supervised machine learning’ and where is it situated within the wider array of AI types?
Vanhove: Instead of a researcher developing hypotheses and picking out a specific set of variables that theory or existing research says should predict the desired outcome, with machine learning the researcher says, “Here are all the variables I have … machine, go ahead and learn from this.” Then, through a series of iterations, the machine will get better and better at weighting or utilizing the variables in different combinations in order to maximize the prediction of the outcome. That’s machine learning in general. There are three different types: 1) unsupervised learning, 2) reinforced learning, and 3) supervised machine learning. We focused our meta-analysis on supervised machine learning because that’s what the vast majority of the existing studies had tested. Now, within the category of supervised machine learning, there are two distinct sub-categories. The first consists of algorithms that are used to predict continuous outcomes. So let’s say age — zero to 100 — is one continuous variable. The other sub-category consists of categorical outcomes, like whether a person left the company or not.
What we ended up finding is that, in the vast majority of the studies we looked at — probably about 100 that we found — 74 were concerned with supervised machine learning to predict categorical outcomes.
CoB: You cite two specific algorithms as performing better than the others. How do you account for that?
Vanhove: We tested eight different algorithms. In the literature, there are two separate dimensions in which they’ve been viewed, and they’re inversely related — as one increases, the other decreases. The first is, “how sophisticated is the algorithm?” That is, how complex is the method it uses to calculate how likely it is, for instance, that someone will leave the company. The other is the computational time it takes for the algorithm to run. We hypothesized that the more sophisticated but also more computationally expensive algorithms were going to perform better. “Boosting” and “Random Forest” fit these criteria, so it wasn’t surprising to us that they performed better relative to the others. The difficulty you run into with sophisticated algorithms, however, is a bit of a black-box problem. With the more sophisticated ones it’s almost always very difficult — and sometimes impossible — to understand the logic behind them and how they develop the probability scores they do. The reason that’s important to human-resources managers is related to the legal defensibility of decision-making. We’re probably a long way from this, but if machine learning were used to make decisions about, say, the hiring of faculty and staff at a large university, and that algorithm is biased or disadvantages people from a protected demographic sub-group, the university could be held liable for employment discrimination. When you don’t know what’s going into an algorithm, and how that leads to the decisions it makes, it’s highly indefensible from a legal point of view. And as far as I know, there’s no current precedent for arguments about the legal defensibility of AI. Everybody just kind of assumes there are going to be big hurdles involved in defending the legality of AI-based decisions, at least in the realm of human-resources management.
CoB: Other thoughts or observations?
Vanhove: I think that as human beings we have a way of getting swept up by the next new thing. As a society we seem to be at that stage right now in terms of AI being this shiny new thing that can eventually benefit mankind. When people hear the term AI now, they’re conditioned to think it isn’t susceptible to the biases of human subjectivity, when in fact it very much is. It’s very much an imperfect tool, and one that right now isn’t in a position to change the way we manage human resources. Yet when people hear the term they tend to think, “O.K., this is better than what we have because it’s objective.” Eventually, maybe it can get there, but I really don’t see AI replacing the human beings who manage human resources. I do see it being beneficial, at some point, in helping human-resources managers do their jobs. I just want to be very clear that we’re not at that point yet.