What Can Artificial Intelligence Offer Humanitarian Mine Action?
CISR JournalThis article is brought to you by the Center for International Stabilization and Recovery (CISR) from issue 28.2 of The Journal of Conventional Weapons Destruction available on the JMU Scholarly Commons and Issuu.com.
An editorial by Russell Gasser, PhD
Would you walk on land declared safe by an unproven technology, developed by enthusiastic proponents who do not have long experience in the world of mine action? What if the system for locating hazards will be tested in only one or two trials, even though the type of machine learning system they are using is known to sometimes give false but completely plausible results (so-called hallucinations1)? Furthermore, in the proposed machine-learning system there will be no audit trail for analysis if a serious error occurs, and no way of knowing for sure how to prevent its repetition.
There is dangerously uncritical promotion on social media of unproven artificial intelligence (AI) technology that is potentially hazardous, insufficiently tested, and unlikely to provide practical solutions in the field. Over twenty years ago, airborne sensors (airship or drone), multi-sensor data fusion, thermal imaging, and many more technologies were promoted as practical solutions for mine clearance, but uptake has been near zero. A drone with sensors linked to an AI system can currently detect a few mine types that are visible on the surface of the ground, over 95 percent of the time. To get from this to near-perfect detection, for unknown mine types including improvised devices, with buried mines and a wide range of different backgrounds, is a monumental task. Separating the different causes of failure such as: sensor limitations, incorrect AI algorithms, or inadequate training data, is a pre-requisite for progress. Standardized AI training data, and defined success criteria agreed by researchers and mine action organizations, are essential if initial trials are to be more than an opportunity to publicize different approaches in carefully prepared scenarios. The use of AI also presents novel legal and liability issues in the event of failure.
The negative consequences of the misuse of machine learning and AI go further than the danger from overlooked hazards. Inappropriate use of AI on safety- critical tasks—especially tasks that humans can already perform to a very high standard—may well prevent AI from being accepted for other uses in mine action where it can make an important difference to the effectiveness and efficiency of operations, and as a result, save lives and prevent injuries. Mine action needs to set out a clear path forward based on understanding of what AI can and cannot provide.
Introduction
AI systems are developing at a breathtaking pace, especially the "Large Language Models" (LLM), like ChatGPT. One of the few certainties is that by the time this article is published, today’s best systems will be overtaken by new developments. AI can now write computer code, translate languages, improve weather forecasting, analyze proteins to find new ways to treat diseases, and far more. AI is set to transform the world in coming years. Correctly applied, it could also bring huge benefits to mine action, which makes the current hyped-up misuse even more disappointing.
But what is AI? And, specifically, what can it contribute to humanitarian mine action (HMA)? This article uses the name AI in the popular sense, not the precise technical meaning—machine learning and generative AI are often confused. The journal New Scientist defines AI as "software used by computers to mimic aspects of human intelligence.”2 The history of computer AI goes back nearly seventy years,3 but three key developments have led to the current extremely powerful LLMs. Firstly, the massive improvement in computer speed, power, and memory over the last five decades coupled with decreased costs. Secondly, the development of the internet and World Wide Web, which give access to the vast amount of information required to train a large general-purpose LLM. And finally, the successful implementation of "multi-layered neural networks" in 2012.4
In practical applications, AI is currently evolving in two different directions. These are not formal categories and they overlap to some degree. The first group is Utility AI, based on specific machine learning to produce assistive tools to help complete tasks. Common examples are digital assistants and language translation programs. In the second group are Generative systems, which use a vast knowledge base to generate new answers in response to carefully crafted questions called prompts. ChatGPT is the best-known example of about fifty large systems currently online in early 2024.
Utility AI Based on Machine Learning
Human users often know the type of result they want from these systems, whether it is converting handwriting to computer text, getting an answer to a spoken question (e.g., using Alexa or Siri), managing complex coordination or logistics, or any one of literally millions of other tasks. The system is expected to deliver a result, not to describe what a range of results might possibly look like. This could help with many organizational tasks within mine action. Current AI could be used to take daily clearance and land release records and return performance and cost analyses, update planning, and generate written reports in different formats, translating them into almost any language and dialect. AI is already used in many industries to support recruitment and training needs, and in mine action, it could deliver the repetitive part of planning and proposal writing, as well as improve coordination. Self-checking by AI, together with human supervision, will still be essential, but nonetheless there are many very useful applications for AI in mine action.
Generative Large Language Models
The second broad category of AI uses general purpose LLMs to provide answers in response to a question or prompt. Writing good prompts, known as prompt engineering, is a new profession that pays very high salaries, even by the standards of the computer industry. The job is lucrative because it requires understanding of neural networks and practical experience of how different LLMs function. It is an illusion to think that anyone from any background can produce ground-breaking results after only a few hours using a public-access LLM. A comparison can be made with chess grand-masters—a new player can learn the rules quickly, but many hours of practice coupled with deep understanding are needed to get the best from the big LLMs.
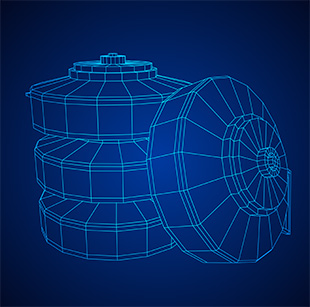
An artificial neural network learns and builds neural pathways based on training in a way that is analogous to the human brain. The largest LLMs currently ingest thousands of gigabytes of information from the internet during training, but even this has its limits. An LLM trained on the sum of human knowledge at earlier stages in human history might insist unequivocally that the sun rotates around the earth, that human sacrifices are necessary to ensure a good harvest, or that slavery is normal and acceptable. Training models cannot escape capturing and potentially exaggerating some systematic errors.
The training sets for large LLMs also rely on human decisions; many hours of human effort are needed to identify, verify, and label information to support training data sets (e.g., manually identifying what can be seen in a photograph by adding tags). This tagging may be outsourced as a low-paid job for people in locations remote from users, maybe in a different cultural context. Time magazine reported that some tagging to identify text snippets was done for ChatGPT by a subcontractor in Kenya and paid less than USD$2 per hour.5,6
Potential Impact
One key area where AI is already having a positive impact globally is in supporting learning and training. Commercial systems are now available (in 2024) to develop training curricula, generate lesson plans, improve testing of learned information and skills, and provide one-to-one support and mentoring to both learners and teachers. Learning experts currently regard the best machine-generated teaching materials as indistinguishable from those prepared by skilled and experienced humans.7 This has obvious application in mine action—especially when linked to machine translation for local languages. The goal is not to ask AI what should be learned, but for humans to define the required knowledge and skills and then get AI to support with the lesson and materials preparation, mentoring for both trainers and trainees, and skills testing methods for certification and quality management.
AI-supported learning could be transformative for explosive ordnance risk education (EORE). A wide range of teaching materials can be generated to deliver the existing, standard, pre-defined messages of EORE in ways that reflect local culture, context, and language, and are compatible with the relevant International Mine Action Standards/National Mine Action Standards and defined national policies. Materials can be updated and adapted quickly, and designed for delivery through different media, in ways that reflect local sensitivities. There is perhaps nothing here that cannot be done by a very large team of skilled and experienced humans, but AI can save a lot of drudge work, and make EORE accessible to a wider audience and to small local organizations who are in touch with hard-to-reach populations. There is no need to wait; this technology is available now in AI learning support systems. It is not specific for mine action and is already being used in workplace training. These systems can also include support for quality management methods such as ISO9001.
The potential application to EORE goes even further. For the first time, AI opens the door to realistic direct checking of likely behavior changes, whether EORE is delivered traditionally or with AI support. An example is the use of apps on cheap smartphones to simulate daily activities (e.g., children on their way to school) and generate behavior prompts from AI-simulated "friends and acquaintances" who encourage either safe or unsafe behavior. The user’s decision can then be used to measure lessons learned. AI can provide a different, unique, and relevant scenario for every child each time it is used, and actively reinforce safe behavior as well as measure success.8
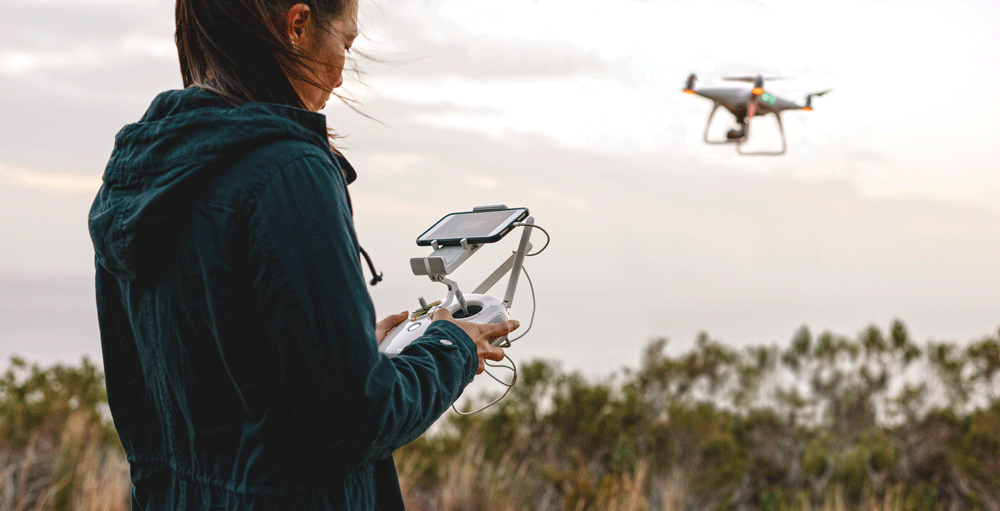
Can We Trust AI Systems?
In a standardized test, different LLMs hallucinated to give entirely wrong but very plausible answers between 3–16 percent of the time.9 Hallucination is a complex issue which leads to an answer that is statistically likely and very convincing, but totally wrong. It is not a cause-and-effect error that can be traced to a single fault. Making mistakes is of course not exclusive to AI—neural networks in the human brain also jump to the wrong conclusions and make obvious errors.10 Self-driving car accident rates demonstrate that even with hundreds of millions of dollars and tens of millions of hours of data collection, some complex systems cannot yet be made 100 percent error-free. We certainly don’t know if it will ever be possible to build a generative large language model that is hallucination free.
For explosive ordnance disposal (EOD) and mine clearance work, we need to know if we can trust AI systems for safety-critical tasks. The internationally-respected journal Nature published an article about "trustworthy AI" in 2022, stating: "As artificial intelligence (AI) transitions from research to deployment, creating the appropriate datasets [...] is increasingly the biggest challenge [...] they critically affect the trustworthiness of the model."11
The key question about trusting neural networks is no longer "do they work?" but rather, “how were they trained?” Two identical AI hardware and software systems with different training data are not at all the same thing, in the same way that two people born on the same day in different places do not have the same experiences and maybe cannot communicate easily with each other. Any proposed safety-critical AI solution for mine action must be defined and evaluated in terms of the training data first and foremost, not just the sensors, hardware, and software. With the exception of a few university and other research departments, training data is a well-guarded commercial secret. Even if data sets were openly described, the sheer size of large model data still prevents analysis; ChatGPT3 was estimated to have used 570 GB of training data, and ChatGPT4 is likely several hundred times larger.12
Conclusions
The first conclusion is positive: machine learning or Utility AI offers significant opportunities for improving effectiveness and efficiency when used in a supporting role in HMA. AI for many applications, including language translation, improving reporting and planning, managing recruitment, and quality management, is now readily available. In particular, current AI already provides the tools to improve all types of training and learning and could transform EORE.
The extremely high cost of developing AI systems means that mine action will be dependent on using and adapting available systems. Any future work should find ways to leverage existing systems to benefit mine action. Collaboration with specialists who have a depth of understanding of and experience in applying AI will be essential.
The training data set is the dominant issue for trustworthy neural networks. LLMs give plausible but completely false answers (hallucination) at low percentage rates, but not zero rates.
Generative AI is unsuitable for safety-critical tasks (e.g., for stand-alone detection and recognition of explosive hazards). Machine learning may well have future application, but any proposed safety-related use should be subjected to rigorous trials scrutinized by independent specialists with expertise in both mine action/EOD and AI systems. The same rigorous safety criteria used for other demining systems (including manual clearance) must be applied.
The ethical implications of AI, including liability in the event of failure, must be considered in parallel with technical and implementation issues. Overall, mine action should seize the opportunity to be more effective and efficient through the use of supportive utility AI. At the same time, bold decision making is needed to categorically reject the wildly popular misapplication of AI technology in safety-related tasks like individual mine detection, until extensive research results demonstrate effectiveness and reliability beyond reasonable doubt.
The author wishes to thank Dr. Robert Keeley for valuable comments on the draft of this article.
See endnotes below.
Russell Gasser, PhD Independent Consultant Russell Gasser, PhD, has worked on new technologies for mine action, results-based and quality management, and evaluation for the European Commission, the Geneva International Centre for Humanitarian Demining (GICHD), the Small Arms Survey, and as an independent consultant. In the late 1990s, he investigated the failure of large-scale research funding to provide matching breakthroughs with new technologies for landmine clearance—the total research funding up to 1998 was nearly US$1 billion. He sees exactly the same exaggerated claims and fundamental errors now being repeated twenty-five years later for drones and AI and hopes that the large-scale allocation of resources to inappropriate techniques will not be repeated.